Can AI Achieve True Creativity?
The criticism that AI cannot create something fundamentally new often overlooks a key fact: human creativity itself is built on combining existing knowledge, concepts, and imagery.
Modern research reveals that when humans invent something novel, the brain doesn’t conjure elements “from nothing” but recombines fragments of prior experiences. Neural networks operate similarly.
When GPT-4 generates text, it relies on statistical patterns learned from existing data rather than conscious intent.
The Brain’s Creative Networks vs. GANs
In one experiment, participants were asked to devise unconventional uses for everyday objects (e.g., a coffee cup). fMRI scans showed two activated networks during creativity:
- The default mode network (associated with daydreaming and associations)
- The frontoparietal network (linked to focus and control)
The dorsolateral prefrontal cortex also activates during musical improvisation. This interaction mirrors generative adversarial networks (GANs), where one component generates ideas and another evaluates their plausibility. Both biological and artificial systems balance freedom and constraints to produce novelty.
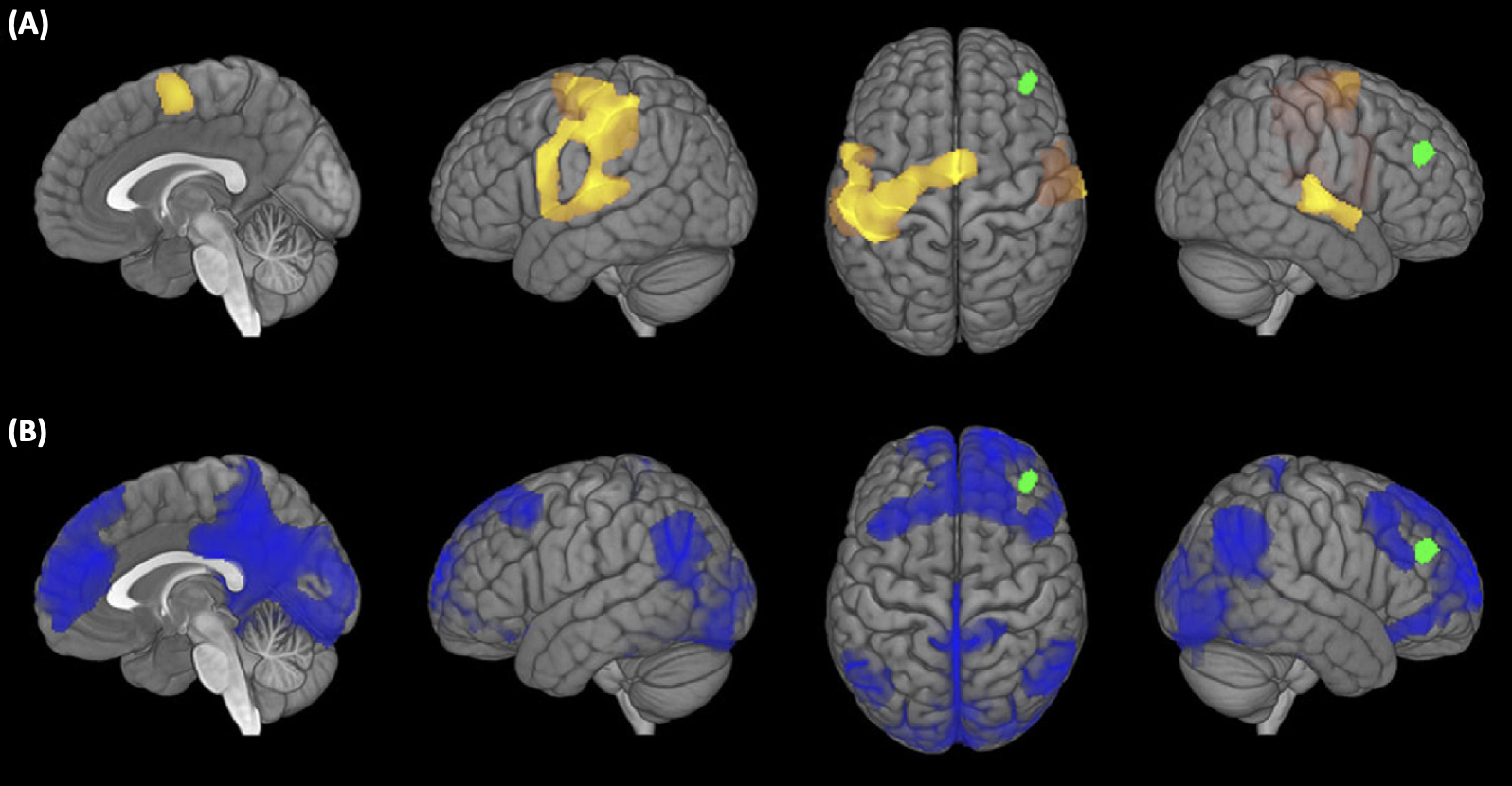
The Limits of Perception and Data
Human perception constrains creativity. We can’t imagine colors beyond the visible spectrum—any new shade is a recombination of known hues. Similarly, Stable Diffusion can’t generate images of objects absent from its training data. It blends learned features, much like the brain combines memories.
Blind individuals describe colors through analogies to sounds or textures (e.g., red as “loud noise,” blue as “smooth surface”). This suggests creativity is rooted in sensory experience—a dimension AI lacks.
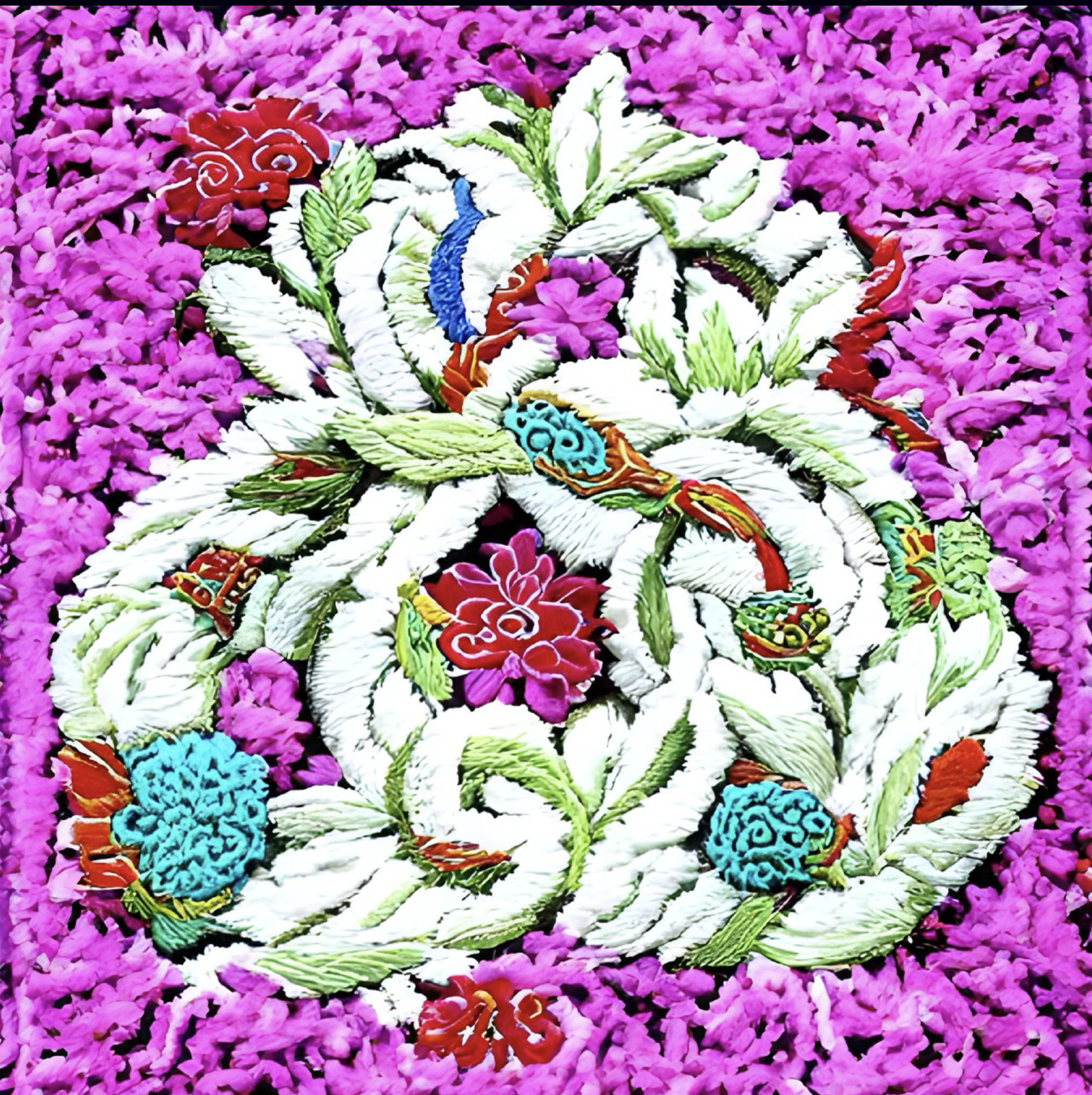
Embodied vs. Abstract Learning
A key difference lies in how humans and AI acquire knowledge:
- Humans learn through physical interaction (e.g., toddlers touching objects to link tactile and visual data)
- AI processes abstract tokens/pixels
While LLMs develop semantic relationships (e.g., “apple” vectors near “fruit” and “tree”), they lack embodied experiences stored in the brain’s sensorimotor cortex. When we think “run,” motor neurons activate—a connection AI can’t replicate.
The Myth of Intentional Creativity
Critics argue humans possess creative “intent,” but jazz improvisation studies show decreased prefrontal cortex activity during spontaneous creation. Ideas emerge automatically from learned patterns—a process strikingly similar to how neural networks operate.
The Originality Debate
If human innovation is recombination, demanding absolute novelty from AI is flawed. Both are constrained by their “training data”:
- Human brains: Biological experiences
- AI models: Digital datasets
The distinction lies in complexity and emotional embodiment. While AI manipulates mathematical structures, the brain ties patterns to emotions and bodily states—for now.
References
[1] Beaty, R. E., Benedek, M., Silvia, P. J., & Schacter, D. L. (2016). Creative Cognition and Brain Network Dynamics. Trends in Cognitive Sciences
[2] De Borst, A. W., & de Gelder, B. (2018). Mental Imagery Follows Similar Cortical Reorganization as Perception: Intra-Modal and Cross-Modal Plasticity in Congenitally Blind. Cerebral Cortex
[3] Mikolov, T., Sutskever, I., Chen, K., Corrado, G. S., & Dea, J. (2013). Distributed Representations of Words and Phrases and their Compositionality
[4] Limb, C. J., & Braun, A. R. (2008). Neural Substrates of Spontaneous Musical Performance: An fMRI Study of Jazz Improvisation
Enjoy Reading This Article?
Here are some more articles you might like to read next: